What is Population Health Analytics?
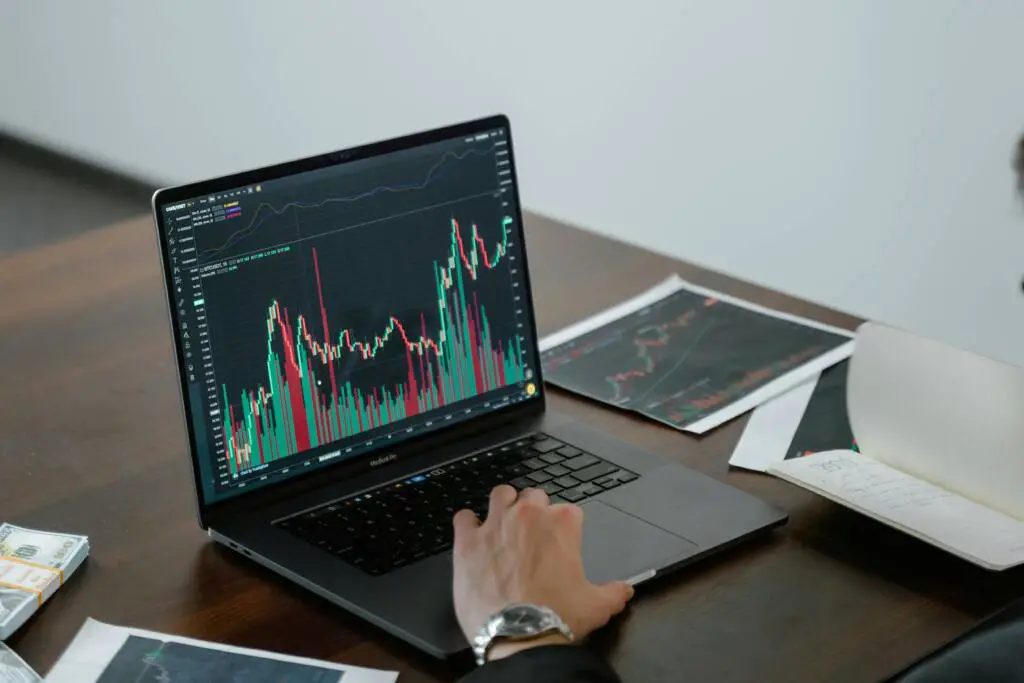
Population health analytics involves analyzing health-related data at a population level. It includes collecting, aggregating, and analyzing data from different sources. The goal is to identify trends, predict and prevent diseases, and improve healthcare outcomes. Healthcare organizations can make better decisions and develop strategies for population health by understanding population health analytics.
What is Population Health Analytics?
Population health analytics is the gathering and analysis of data to understand the health status, trends, and causes of a particular population. Healthcare providers can use statistical methods, data mining, machine learning, and other techniques to find useful information from big datasets. By using population health analytics, providers can understand the health needs and risks of individuals in a population. This helps them deliver more targeted and effective care.
Why is Population Health Analytics Important?
The need for population health analytics stems from the desire to improve healthcare outcomes and reduce healthcare costs. By understanding the health trends and factors that influence a population’s health, healthcare organizations can develop targeted interventions, allocate resources more effectively, and deliver proactive care. Let’s delve into some key reasons why population health analytics is important in healthcare.
Identifying health trends in a population: Population health analytics helps healthcare organizations identify patterns and trends in a population’s health. By analyzing data on demographics, social determinants of health, and health outcomes, healthcare providers can identify high-risk groups, prevalent diseases, and factors that contribute to poor health. This information allows them to develop interventions and allocate resources accordingly.
Predicting and preventing diseases: Population health analytics enables healthcare professionals to predict and prevent diseases by using predictive modeling techniques. By analyzing data on factors such as genetics, lifestyle choices, and environmental exposures, healthcare organizations can identify individuals at high risk of developing certain conditions and provide targeted interventions to prevent disease progression.
Improving healthcare outcomes: Population health analytics plays a crucial role in improving healthcare outcomes. By analyzing data on healthcare utilization, treatment outcomes, and patient satisfaction, healthcare organizations can identify areas for improvement and implement evidence-based interventions. This proactive approach leads to better patient outcomes, reduced hospital readmissions, and improved overall population health.
How Does Population Health Analytics Work?
Population health analytics involves several distinct steps, each contributing to a comprehensive understanding of population health. Let’s explore the process of population health analytics in more detail.
Data collection and aggregation: The first step in population health analytics is the collection and aggregation of data from various sources. These sources may include electronic health records, insurance claims, public health databases, and social determinants of health. By consolidating these diverse datasets, healthcare organizations can create a comprehensive picture of the population’s health profile.
Data analysis and visualization: Once the data is collected and aggregated, the next step is to analyze and visualize it. Data analysis involves using statistical models and machine learning algorithms to identify patterns, relationships, and trends in the data. Visualization tools, such as dashboards and heat maps, help present the findings in a visually appealing and understandable format, allowing healthcare professionals to grasp the key insights quickly.
Insights and decision-making: Healthcare organizations use the insights gleaned from data analysis to identify areas for improvement, develop targeted interventions, and allocate resources efficiently. These data-informed decisions contribute to better healthcare outcomes and improved population health.
Benefits of Population Health Analytics
Population health analytics offers several advantages that can help healthcare organizations enhance their operations and improve patient care.
Let’s explore some of the benefits:
Proactive healthcare management: By analyzing population health data, healthcare organizations can proactively identify individuals at high risk for diseases or adverse health outcomes. This allows them to intervene early, provide preventive care, and manage chronic conditions more effectively. Proactive healthcare management improves patient outcomes and reduces healthcare costs.
Cost-effective healthcare strategies: Population health analytics also helps healthcare organizations develop cost-effective strategies. By identifying high-risk groups and prevalent diseases, healthcare providers can allocate resources and interventions where they are most needed. This targeted approach optimizes resource utilization and reduces unnecessary healthcare expenditures.
Enhanced patient outcomes: One of the primary goals of population health analytics is to improve patient outcomes. By analyzing data on treatment outcomes, healthcare utilization, and patient satisfaction, healthcare organizations can identify areas for improvement and implement evidence-based interventions. As a result, patient outcomes improve, hospital readmissions decrease, and overall population health is enhanced.
Types of Data Used
https://7thavewellnessblog.com/?p=3799
To gain a comprehensive understanding of population health, healthcare organizations collect and analyze various types of data. Let’s explore some of the different types of data sources commonly used in population health analytics.
Electronic health records: Electronic health records (EHRs) contain valuable clinical information about individuals, including medical history, diagnoses, medications, and laboratory results. Accessing and analyzing EHR data allows healthcare organizations to gain insights into disease prevalence, treatment outcomes, and healthcare utilization patterns.
Social determinants of health: Social determinants of health are the social, economic, and environmental factors that affect an individual’s health. These factors include socioeconomic status, education level, employment status, access to healthcare, and neighborhood conditions. By incorporating data on social determinants of health, healthcare organizations can understand the broader context in which individuals live and tailor interventions accordingly.
Behavioral and lifestyle data: Behavioral and lifestyle data provide valuable insights into individuals’ habits, choices, and preferences. These data sources include surveys, wearable devices, and mobile applications that track physical activity, nutrition, sleep patterns, and other lifestyle factors. By collecting and analyzing behavioral and lifestyle data, healthcare organizations can develop interventions and preventive strategies that target specific behaviors and improve overall health outcomes.
Tools and Techniques
To effectively analyze and interpret population health data, healthcare organizations rely on a variety of tools and techniques. Let’s explore some of the popular tools and techniques used in population health analytics.
Data mining and machine learning: Data mining and machine learning techniques are used to identify patterns, correlations, and relationships within large datasets. These techniques help uncover hidden insights and predictive models that can be used to inform interventions and improve healthcare outcomes.
Predictive modeling: Predictive modeling uses historical data to predict future health outcomes or events. By leveraging algorithms and statistical models, healthcare organizations can identify individuals at high risk for certain diseases or adverse outcomes. This allows them to provide targeted interventions, preventive care, and early interventions to improve patient outcomes.
Risk stratification: Risk stratification involves dividing a population into different risk groups based on their likelihood of developing certain diseases or experiencing adverse health events. By stratifying the population, healthcare organizations can allocate resources and interventions more effectively, focusing on those at the highest risk. This approach optimizes resource utilization and improves population health outcomes.
Challenges and Limitations
Like any field, this one also comes with its fair share of challenges and limitations. Understanding these challenges is crucial in developing effective population health analytics strategies. Let’s explore some of the challenges faced in population health analytics.
Data privacy and security: One of the primary challenges in population health analytics is ensuring data privacy and security. With the vast amount of health data being collected and analyzed, it is essential to protect individuals’ personal health information from unauthorized access or breaches. Healthcare organizations must implement robust data security measures and adhere to strict privacy regulations to maintain patient trust.
Data interoperability issues: Another challenge in population health analytics is data interoperability. Healthcare organizations often have data stored in various systems and formats, making it challenging to integrate and analyze the data seamlessly. Achieving interoperability requires the adoption of standards and interoperability frameworks to facilitate the exchange and analysis of data across different systems.
Ethical considerations: There are ethical considerations to be aware of in population health analytics. The use of personal health data raises concerns regarding consent, data ownership, and the potential for bias or discrimination. Healthcare organizations must navigate these ethical considerations carefully, ensuring that data collection and analysis are conducted responsibly and transparently.
Best Practices
Healthcare organizations can maximize the effectiveness of population health analytics by following best practices. These strategies ensure accurate data analysis, effective interventions, and improved population health outcomes.
Let’s explore some of the best practices:
Collaborative data sharing: Collaborative data sharing promotes information exchange and collaboration among healthcare organizations, researchers, and policymakers. By sharing data, organizations can pool resources, gain access to larger datasets, and derive more comprehensive insights for population health management.
Continuous evaluation and improvement: Population health analytics is an ongoing process that requires continuous evaluation and improvement. Healthcare organizations should regularly assess the effectiveness of their interventions, identify areas for improvement, and adjust their strategies accordingly. It is essential to monitor key performance indicators and outcomes to ensure that population health goals are being met.
Engaging stakeholders: Engaging stakeholders, including healthcare providers, patients, community organizations, and policymakers, is vital for successful population health analytics. Stakeholder engagement ensures that the data collected and analyzed are relevant, meaningful, and aligned with the needs and goals of the population. Engaging stakeholders also fosters a collaborative approach to population health management and encourages the adoption of data-driven interventions.
Real-World Examples
Several real-world examples demonstrate the successful implementation of population health analytics and the impact it can have on healthcare outcomes. Let’s explore some case studies that highlight the benefits of population health analytics.
Reducing chronic disease prevalence: One example of successful population health analytics is the reduction of chronic disease prevalence. By analyzing data on risk factors, healthcare utilization, and treatment outcomes, healthcare organizations can develop preventive strategies and targeted interventions to reduce the prevalence of chronic diseases like diabetes and hypertension. This leads to healthier populations, improved quality of life, and reduced healthcare costs.
Targeted interventions for vulnerable populations: Another example is the use of population health analytics to develop targeted interventions for vulnerable populations. By analyzing data on social determinants of health, healthcare organizations can identify individuals or communities at high risk of poor health outcomes. This allows them to direct resources and interventions to address the unique challenges faced by these populations, reducing health disparities and improving health equity.
Improving healthcare resource allocation: By analyzing data on healthcare utilization, hospital readmissions, and emergency department visits, healthcare organizations can identify areas with high healthcare demand and redistribute resources accordingly. This ensures that healthcare resources are allocated where they are most needed, improving access to care and optimizing healthcare delivery.
Future Trends
As the field of population health analytics continues to evolve, several emerging trends and future directions can be expected to shape its development. Let’s explore some of the future trends in population health analytics.
Integration of wearables and IoT devices: Wearables and IoT devices will be important. These devices collect real-time data on people’s health and activity. Healthcare organizations can use this data to better understand people’s health and offer customized interventions.
Precision medicine and personalized care: Population health analytics will likely use precision medicine and personalized care more. By analyzing genetic data, biomarkers, and clinical information, healthcare organizations can make personalized treatment plans. These plans consider individuals’ unique characteristics and health needs. Precision medicine and personalized care can improve treatment outcomes and reduce healthcare costs.
Artificial intelligence and advanced analytics: AI algorithms can analyze lots of data, find complex patterns, and make predictive models. This helps with accurate risk stratification, disease prediction, and treatment optimization. Advanced analytics techniques like natural language processing and deep learning can get important information from unstructured data sources like medical journals and patient narratives.
https://www.ncbi.nlm.nih.gov/
Wrap Up
Population health analytics is important for improving healthcare outcomes. It analyzes data at a population level. Understanding the basics of population health analytics, its importance, tools, and best practices helps healthcare organizations make data-informed decisions. They can also develop proactive strategies to enhance population health. Stay tuned for future advancements and innovations in this rapidly evolving field.
FAQ’s
Q: What is population health analytics?
A: Population health analytics is the use of data analytics to improve population health. It involves analyzing vast amounts of data related to the entire population to identify health concerns, address social determinants of health, and provide actionable insights for healthcare providers to make informed decisions in improving the quality of care and patient experience.
Q: How does population health analytics benefit the healthcare system?
A: Population health analytics provides healthcare solutions for population health management by enabling health systems and health plans to invest in using data to identify risk profiles, manage care needs, and enable proactive and personalized care delivery. It helps in chronic disease management, patient engagement, and addressing social determinants of health.
Q: What kind of data is used in population health analytics?
A: Population health analytics utilizes both payer data, such as claims data, and clinical data to provide insights into the quality of care, patient outcomes, and cost of care. This data is used to create risk profiles, identify gaps in care management, and provide evidence-based recommendations for improving population health.
Q: How does population health analytics help in improving patient care?
A: By analyzing data related to patient care, population health analytics helps healthcare providers make informed decisions about care management, engage patients in their own health, and identify interventions to improve patient outcomes and satisfaction. It also enables the identification of trends and patterns in patient populations, leading to more effective and personalized care delivery.
Q: What role does population health analytics play in value-based care?
A: Population health analytics plays a crucial role in value-based care by providing insights into the quality and cost-effectiveness of care delivery. It helps in identifying areas for improvement, measuring the impact of interventions, and aligning incentives with performance. Thus driving the transition toward value-based care models.